Decision Analysis for Management Judgment,
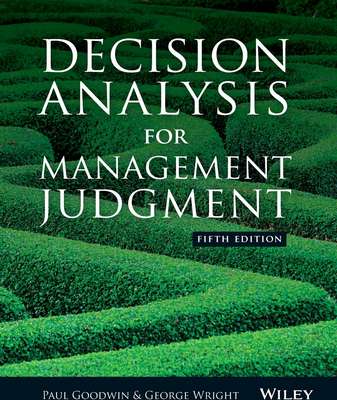
Námskeið
- T-603 Ákvarðanatökuaðferðir.
Ensk lýsing:
Decision Analysis for Management Judgment is unique in its breadth of coverage of decision analysis methods. It covers both the psychological problems that are associated with unaided managerial decision making and the decision analysis methods designed to overcome them. It is presented and explained in a clear, straightforward manner without using mathematical notation. This latest edition has been fully revised and updated and includes a number of changes to reflect the latest developments in the field.
Lýsing:
Decision Analysis for Management Judgment is unique in its breadth of coverage of decision analysis methods. It covers both the psychological problems that are associated with unaided managerial decision making and the decision analysis methods designed to overcome them. It is presented and explained in a clear, straightforward manner without using mathematical notation. This latest edition has been fully revised and updated and includes a number of changes to reflect the latest developments in the field.
Annað
- Höfundar: Paul Goodwin, George Wright
- Útgáfa:5
- Útgáfudagur: 2014-04-28
- Hægt að prenta út 10 bls.
- Hægt að afrita 2 bls.
- Format:ePub
- ISBN 13: 9781118887875
- Print ISBN: 9781118740736
- ISBN 10: 1118887875
Efnisyfirlit
- Front Matter
- Contents
- Foreword
- Preface
- Accompanying website at http://www.wiley.com/college/goodwin/
- Complex decisions
- The role of decision analysis
- Good and bad decisions and outcomes
- Applications of decision analysis
- Improved strategic decision making at Du Pont8
- Structuring decision problems in the International Chernobyl Project9, 10
- Selecting research projects at a large international pharmaceutical company11
- Petroleum exploration decisions at the Phillips Petroleum Company12
- Prioritizing infrastructure-renewal projects at MIT13
- Supporting the systems-acquisition process for the US military14
- Prioritizing projects in a busy UK social services department15
- Selecting a wide area network solution at EXEL Logistics16
- Planning under a range of futures in a financial services firm
- Supporting top-level political decision making in Finland17
- Automating advice-giving in a building society front office
- Allocating funds between competing aims in a shampoo-manufacturing company18
- Anticipating the need for doctors and dentists in the English National Health Service19
- Monitoring early warning signals in the business environment at Nokia and Statoil20
- The future of electric-drive vehicles in Germany21
- Overview of the book
- References
- Introduction
- Heuristics used for decisions involving multiple objectives
- The recognition heuristic
- The minimalist strategy2
- Take the last2
- The lexicographic strategy8
- The semi-lexicographic strategy8
- Elimination by aspects12
- Sequential decision making: satisficing
- Reason-based choice
- Factors affecting which strategies people employ
- Other characteristics of decision making involving multiple objectives
- Decoy effects22
- Choosing by unique attributes
- Emotion and choice
- Justifying already-made choices
- Partitioning the total cost of an item changes preferences
- Summary
- Discussion questions
- References
- Introduction
- Basic terminology
- Objectives and attributes
- Value and utility
- An office location problem
- An overview of the analysis
- Constructing a value tree
- Figure 3.1 – A value tree for the office location problem
- Measuring how well the options perform on each attribute
- Table 3.1 – Costs associated with the seven offices
- Direct rating
- Figure 3.2 – A value scale for office image
- Table 3.2 – Values and weights for the office location problem
- Value functions
- Figure 3.3 – Constructing a value function for office floor area
- Figure 3.4 – A value function for distance from customers
- Figure 3.5 – Derivation of swing weights. For example, a swing from the worst to the best location for visibility is considered to be 80% as important as a swing from the worst to the best location for closeness to customers
- Figure 3.6 – A plot of benefits against costs for the seven offices
- Figure 3.7 – Sensitivity analysis for weight placed on image
- The axioms of the method
- Assumptions made when aggregating values
- Value-focused thinking
- Exercises
- Introduction
- SMARTER
- Table 4.1 – ROC weights
- Even Swaps
- Even Swaps versus SMART
- The relative strengths of Even Swaps
- The relative limitations of Even Swaps
- The analytic hierarchy process
- Figure 4.1 – A hierarchy for the packaging machine problem
- Table 4.2 – Comparing the importance of ‘Costs’ and ‘Quality’
- Table 4.3 – Comparing the importance of the ‘Quality’ attributes
- Table 4.4 – Comparing the machines on ‘purchase cost’
- Figure 4.2 – Weights for the packaging machine problem
- Performing AHP calculations by hand
- Table 4.5 – Random indices for checking the consistency of a table
- The axioms of the AHP
- The AHP versus SMART
- The relative strengths of the AHP
- Criticisms of the AHP
- MACBETH
- Summary
- Exercises
- References
- Introduction
- Outcomes and events
- Approaches to probability
- The classical approach
- The relative frequency approach
- The subjective approach
- Mutually exclusive and exhaustive events
- The addition rule
- Table 5.1 – The frequency of flooding of a tidal river in April over the last 20 years
- The addition rule
- Table 5.2 – Results of a survey of workers in a branch of the chemicals industry
- Figure 5.1 – A probability tree
- Figure 5.2 – Probability distribution for the number of planes in service by the end of the year
- Figure 5.3 – Probability distribution for project completion time
- Figure 5.4 – Cumulative distribution function for project completion time
- Exercises
- Introduction
- The maximin criterion
- Table 6.1 – A decision table for the food manufacturer
- The expected monetary value criterion
- Table 6.2 – Another decision table for the food manufacturer
- Figure 6.1 – A sensitivity analysis for the food manufacturer’s problem
- Limitations of the EMV criterion
- Table 6.3 – Returns and probabilities for the new component problem
- Single-attribute utility
- Figure 6.2 – A decision tree for the conference organizer’s problem
- Figure 6.3 – The conference organizer’s decision tree with utilities
- Figure 6.4 – A demonstration of how expected utility reduces the decision to a simple choice between lotteries
- Interpreting utility functions
- Figure 6.5 – A utility function for the conference organizer
- Figure 6.6 – Interpreting the shape of a utility function
- Utility functions for non-monetary attributes
- Figure 6.7 – A decision tree for the drug company research department problem
- Figure 6.8 – A utility function for product development time
- The axioms of utility
- Figure 6.9 – The continuity axiom
- Figure 6.10 – The substitution axiom
- Figure 6.11 – Demonstration of the substitution axiom
- Figure 6.12 – Demonstration of the unequal-probability axiom
- Figure 6.13 – Demonstration of the compound-lottery axiom
- More on utility elicitation
- How useful is utility in practice?
- Figure 6.14 – Allais’s paradox
- Multi-attribute utility
- The Decanal Engineering Corporation
- Figure 6.15 – A decision tree for the project manager’s problem
- Mutual utility independence
- Figure 6.16 – Determining utility independence
- Deriving the multi-attribute utility function
- Stage 1
- Figure 6.17 – Utility functions for overrun time and project cost
- Table 6.4 – The project manager’s utilities for overrun and cost
- Stage 2
- Figure 6.18 – Determining k1
- Figure 6.19 – Determining k2
- Figure 6.20 – The project manager’s decision tree with utilities
- Stage 3
- Figure 6.21 – Checking the consistency of the decision maker’s responses
- Stage 1
- The Decanal Engineering Corporation
- Interpreting multi-attribute utilities
- Further points on multi-attribute utility
- Exercises
- Introduction
- Constructing a decision tree
- Figure 7.1 – An initial decision tree for the food-processor problem
- Figure 7.2 – A new decision tree for the food-processor problem
- Determining the optimal policy
- Figure 7.3 – Rolling back the decision tree
- Decision trees and utility
- Figure 7.4 – The engineer’s utility function
- Figure 7.5 – Apply the rollback method to a decision tree involving utilities
- Decision trees involving continuous probability distributions
- Figure 7.6 – The extended Pearson–Tukey (EP-T) approximation method
- Assessment of decision structure
- Figure 7.7 – One decision-analytic representation of the calculator problem
- Figure 7.8 – Toward the correct decision-analytic representation of the calculator problem?
- Figure 7.9 – Phases of a decision analysis
- Figure 7.10 – A possible fault tree for discovering why a car will not start (adapted from Fischhoff, B., Slovic, P. and Lichtenstein (1978) Fault Trees: Sensitivity of Estimated Failure Probabilities to Problem Representation, Journal of Experimental Psychology: Human Perception and Performance, 4(2), 330–344. Copyright 1978 © American Psychological Association. By permission of the authors)
- Eliciting decision-tree representations
- Figure 7.11 – Definitions used in influence diagrams
- Figure 7.12 – Influence diagram
- Figure 7.13 – Decision tree derived from influence diagram
- Summary
- Exercises
- References
- Introduction
- Monte Carlo simulation
- Table 8.1 – Ten simulations of monthly cash flows
- Table 8.2 – Estimating probabilities from the simulation results
- Table 8.3 – The effect of the number of simulations on the reliability of the probability estimates
- Applying simulation to a decision problem
- The Elite Pottery Company
- Stage 1: Identify the factors
- Figure 8.1 – Identifying the factors that will affect the profit earned by the commemorative plate
- Stage 2: Formulate a model
- Stage 3: Preliminary sensitivity analysis
- Table 8.4 – Estimates of lowest, highest and most likely values for the Elite Pottery problem
- Figure 8.2 – Tornado diagram showing the effect on profit if each factor changes from its lowest to its highest possible value
- Stage 4: Assess probability distributions
- Figure 8.3 – Probability distributions for variable costs, sales and fixed costs
- Stage 5: Perform the simulation
- Figure 8.4 – Probability distribution for profit earned by the commemorative plate
- Stage 6: Sensitivity analysis on the results of the simulation
- Stage 7: Compare alternative course of action
- Stage 1: Identify the factors
- Plotting the two distributions
- Figure 8.5 – A comparison of the profit probability distributions of the commemorative plate and the figurine
- Determining the option with the highest expected utility
- Stochastic dominance
- First-order stochastic dominance
- Figure 8.6 – First-order stochastic dominance
- Second-order stochastic dominance
- Figure 8.7 – Second-order stochastic dominance
- Figure 8.8 – An example where the test for second-order stochastic dominance is inconclusive
- First-order stochastic dominance
- The Elite Pottery Company
- The mean–standard deviation approach
- Figure 8.9 – The mean–standard deviation screening method
- Figure 8.10 – (a) A normal probability distribution for profit; (b) examples of quadratic utility functions
- The NPV method
- Table 8.5 – Calculating the NPVs for the Alpha and Beta machines
- Using simulation
- Figure 8.11 – Probability distributions for the Alpha machine (vertical axes represent probability density)
- Figure 8.12 – Probability distributions for the NPVs of the Alpha and Beta machines
- Utility and net present value
- Exercises
- Introduction
- Bayes’ theorem
- Figure 9.1 – Tree diagram for the components problem
- Figure 9.2 – Applying Bayes’ theorem to the components problem
- Example
- Answer
- Figure 9.3 – Applying Bayes’ theorem to the equipment operating problem
- Another example
- Figure 9.4 – Applying Bayes’ theorem to the sales manager’s problem
- Figure 9.5 – The effect of vague prior probabilities and very reliable information
- Figure 9.6 – The effect of the reliability of new information on the modification of prior probabilities for the gas-exploration problem
- Figure 9.7 – (a) A decision tree for the retailer’s problem based on prior probabilities; (b) applying Bayes’ theorem to the retailer’s problem; (c) a decision tree for the retailer’s problem using posterior probabilities
- The expected value of perfect information
- Figure 9.8 – Determining the expected value of perfect information
- Table 9.1 – Calculating the expected value of perfect information
- The expected value of imperfect information
- Figure 9.9 – Deciding whether or not to buy imperfect information
- Figure 9.10 – (a) Revising the prior probabilities when the test indicates that the virus is present; (b) revising the prior probabilities when the test indicates that the virus is absent
- Figure 9.11 – Determining the expected value of imperfect information
- Exercises
- Introduction
- Test your judgment
- Heuristics and biases
- The availability heuristic
- Biases associated with the availability heuristic
- 1. When ease of recall is not associated with probability
- 2. Ease of imagination is not related to probability
- Test your judgment: answers to questions 1 and 2
- 3. Illusory correlation
- Biases associated with the availability heuristic
- Biases associated with the representativeness heuristic
- 1. Ignoring base-rate frequencies
- Test your judgment: answer to question 3
- 2. Expecting sequences of events to appear random
- Test your judgment: answer to question 4
- 3. Expecting chance to be self-correcting
- Test your judgment: answers to questions 5 and 6
- 4. Ignoring regression to the mean
- Test your judgment: answer to question 7
- 5. The conjunction fallacy
- Test your judgment: answers to questions 8 and 9
- 1. Ignoring base-rate frequencies
- Biases associated with anchoring and adjustment
- 1. Insufficient adjustment
- Test your judgment: answer to question 10
- 2. Overestimating the probability of conjunctive events
- Test your judgment: answer to question 11
- 3. Underestimating probabilities for disjunctive events
- Test your judgment: answer to question 12
- 3. Overconfidence
- Test your judgment: answer to question 13
- 1. Insufficient adjustment
- 1. Believing desirable outcomes are more probable
- 2. Biased assessment of covariation
- Test your judgment: answer to question 14
- 1. Participants in studies may be unrepresentative of real decision makers
- 2. Laboratory tasks may be untypical of real-world problems
- 3. Experimental tasks may be understood in different ways by participants
- 4. Participants may be poorly motivated
- 5. Citation bias
- 6. Real-world studies suggest better performance
- 7. People think in terms of frequencies not probabilities
- Figure 10.1 – A methodology for choosing how to develop a subjective probability assessment
- Exercises
- Introduction
- Probability assessment
- Issues with verbal probability expressions
- Coherence in probability judgments
- Two barriers to improving probability assessments through learning
- Preparing for probability assessment
- Motivating
- Structuring
- Conditioning
- Assessment methods
- Assessment methods for individual probabilities
- Direct assessments
- The probability wheel
- Figure 11.1 – A probability wheel
- Assessment methods for individual probabilities
- Assessment methods for probability distributions
- The probability method
- Graph drawing
- Figure 11.2 – The method of relative heights
- Figure 11.3 – Calibration curves
- Event trees
- Figure 11.4 – An event tree
- Fault trees
- Figure 11.5 – A fault tree
- Using a log-odds scale
- Figure 11.6 – A log-odds scale
- Exercises
- Introduction
- The Two Valleys Company
- Exploring sources of uncertainty
- Figure 12.1 – Sources of uncertainty at the Two Valleys Company
- Table 12.1 – Estimated values for uncertain factors
- Figure 12.2 – Cumulative probability distributions for annual profit at the two sites
- Identifying possible areas for uncertainty management
- 1. Calculate the effect of perfect control
- Table 12.2 – Results of risk management actions
- Figure 12.3 – Tornado diagram for Littleton
- 2. Repeat the above process for the next best option
- Figure 12.4 – Tornado diagram for Callum Falls
- 1. Calculate the effect of perfect control
- Exploring sources of uncertainty
- Using brainstorming to create actions to improve the preferred policy
- Table 12.3 – Ideas for risk management at Two Valleys Company
- Figure 12.5 – The effectiveness of risk management measures
- Exercise
- Figure 12.6 – Cumulative probability distribution for the AB Charity
- Figure 12.7 – Tornado diagram for the AB Charity
- Introduction
- Mathematical aggregation
- Table 13.1 – The production manager’s utilities and probabilities
- Table 13.2 – The accountant’s utilities and probabilities
- Table 13.3 – The average of the utilities and probabilities
- Aggregating judgments in general
- Taking a simple average of the individual judgments
- Taking a weighted average of the individual judgments
- Aggregating probability judgments
- Table 13.4 – Averaging probabilities
- Aggregating preference judgments
- Aggregating preference orderings
- Aggregating values and utilities
- Figure 13.1 – Measuring individuals’ strengths of preference against a common scale
- Figure 13.2 – The accuracy of the median estimate
- The benefits of establishing heterogeneity in Delphi panels
- Enhancing the evaluation of rationales by devil’s advocacy and dialectical inquiry
- Discussion questions
- Introduction
- Modeling resource allocation problems
- An illustrative problem
- The main stages of the analysis
- Determining the areas, resources and benefits5
- Identifying the possible strategies for each region
- Figure 14.1 – Possible strategies identified by managers of the furniture company
- Assessing the costs and benefits of each strategy
- Table 14.1 – Values of the strategies in the individual regions
- Measuring each benefit on a common scale
- Figure 14.2 – The within-criterion weights for the furniture company problem
- Table 14.2 – Values of strategies with each benefit measured on a common scale
- Comparing the relative importance of the benefits
- Figure 14.3 – The across-criteria weights for the furniture company problem
- Identifying the costs and benefits of the packages
- Figure 14.4 – Identifying the efficient frontier for the furniture company problem
- Figure 14.5 – Investigating the costs and benefits of strategies in the individual regions
- Figure 14.6 – Management and union value functions and weights
- Table 14.3 – Calculation of values for the tentative management–union deal
- Figure 14.7 – Identifying the efficient frontier for the management–union negotiations
- Discussion questions
- Table 14.4 – Details of deals
- Figure 14.8 – Values of deals
- Introduction
- Creativity in problem solving
- Figure 15.1 – Moves to attain the goal state in the first water-jug problem
- Figure 15.2 – Moves to attain the goal state in the second water-jug problem
- Figure 15.3 – The nine-dot problem
- How people frame decisions
- Solving the wrong problem
- Get hooked on complexity – overlooking simple options
- Imposing imaginary constraints and false assumptions on the range of options
- Sensitivity to reference points
- Prospect theory
- Figure 15.4 – Value function in prospect theory
- Figure 15.5 – Decision weights in prospect theory
- Figure 15.6 – Choice between alternatives P and Q
- Figure 15.7 – P and Q with values and decision weights replacing original numbers
- Prospect theory
- Real-world studies
- Studies in the psychological laboratory
- Figure 15.8 – An example of a response to Russo and Schoemaker’s frame analysis questions
- Figure 15.9 – The relationship between the perceived business environment and the strategic decision process
- Discussion questions
- Figure 15.10 – Solution to the nine-dot problem (Figure 15.3)
- Introduction
- Figure 16.1 – The Müller–Lyer illusion
- Scenario construction: the extreme-world method
- Figure 16.2 – Steps in scenario construction: the extreme-world method
- Figure 16.3 – Predetermined trends
- Figure 16.4 – Key uncertainties
- Figure 16.5 – Three scenarios
- Using scenarios in decision making
- Figure 16.6 – An illustrative business idea for a business school
- Figure 16.7 – Testing the robustness of strategies against scenarios
- Figure 16.8 – A ‘real’ scenario of future trading patterns
- Scenario construction: the driving forces method
- Figure 16.9 – An output of a ‘driving forces’ scenario structuring methodology
- Figure 16.10 – Adam Kahane’s four South African scenarios
- Figure 16.11 – Steps in scenario construction: the driving force method
- Figure 16.12 – Stakeholder structuring space
- Case study of a scenario intervention in the English National Health Service6
- Case study of a scenario intervention in the public sector7
- Forward to the past
- Free enterprise
- People’s kailyard8
- Technology serves
- Table 16.1 – The components of the futures methodologies
- Organizational context and scenario planning
- Case study of an unsuccessful scenario-planning intervention
- Dealing with low predictability
- Discussion questions
- Introduction
- Main stages of the approach
- Table 17.1 – Stages in the application of SMART to scenario planning
- Illustrative case study
- Stage 1: Formulate objectives
- Stage 2: Formulate scenarios
- Stage 3: Design alternative strategies
- Stage 4
- Table 17.2 – Strategy ranks across different scenarios for each objective (1 = best)
- Table 17.3 – Scores for strategies under the different scenarios for each objective
- Stage 5
- Table 17.4 – Ranking of swings
- Table 17.5 – Obtaining the weights for the objectives
- Stage 6: For each strategy/scenario combination use the performance scores and weights to determine a weighted aggregate score
- Table 17.6 – Obtaining an aggregate score for Status Quo in the Mail Mountain scenario
- Stage 7: Produce a table of strategy/scenario aggregate scores and use this to assess and compare the strategies’ performances, paying particular attention to the robustness of performance over the range of scenarios
- Table 17.7 – Aggregate scores
- Stage 8: Perform sensitivity analysis
- Table 17.8 – The effect of changing the weight on growth
- Having a checklist of key objectives
- Making the ranking process easier
- Avoiding the need to assign scores and weights
- Different weights for different scenarios
- Other approaches
- Exercises
- Introduction
- Expert systems
- What is an expert system?
- What is expert knowledge?
- How is expert knowledge represented in expert systems?
- Marketing applications
- Financial services applications
- Figure 18.1 – Underwriting options
- Figure 18.2 – The rule base of the geographical module
- Where next?
- Back-office fraud-detection systems
- Point-of-sale advice-giving systems
- Conclusion
- Statistical models of judgment
- If linear models are so effective, why aren’t they more prevalent in practice?
- Comparisons of decision-aiding techniques
- Snap decisions and decision analysis: why not trust our initial intuitions?
- Designing decisions so that people make the ‘best’ choice
- Some final words of advice
- Are my assumptions about the business environment and the future valid?
- Who should I involve in the decision?
- Have I made an adequate search for alternative courses of action?
- How much effort is the decision worth? Which aspects of the problem require the most effort?
- Which method(s) are likely to help with my decision problem?
- Table 18.1 – Summary of techniques covered in the book
- Suggested answers to selected questions
- Chapter 2
- Chapter 3
- Chapter 4
- Chapter 5
- Chapter 6
- Chapter 7
- Chapter 8
- Chapter 9
- Chapter 11
- Chapter 14
- Chapter 17
- Index
UM RAFBÆKUR Á HEIMKAUP.IS
Bókahillan þín er þitt svæði og þar eru bækurnar þínar geymdar. Þú kemst í bókahilluna þína hvar og hvenær sem er í tölvu eða snjalltæki. Einfalt og þægilegt!Rafbók til eignar
Rafbók til eignar þarf að hlaða niður á þau tæki sem þú vilt nota innan eins árs frá því bókin er keypt.
Þú kemst í bækurnar hvar sem er
Þú getur nálgast allar raf(skóla)bækurnar þínar á einu augabragði, hvar og hvenær sem er í bókahillunni þinni. Engin taska, enginn kyndill og ekkert vesen (hvað þá yfirvigt).
Auðvelt að fletta og leita
Þú getur flakkað milli síðna og kafla eins og þér hentar best og farið beint í ákveðna kafla úr efnisyfirlitinu. Í leitinni finnur þú orð, kafla eða síður í einum smelli.
Glósur og yfirstrikanir
Þú getur auðkennt textabrot með mismunandi litum og skrifað glósur að vild í rafbókina. Þú getur jafnvel séð glósur og yfirstrikanir hjá bekkjarsystkinum og kennara ef þeir leyfa það. Allt á einum stað.
Hvað viltu sjá? / Þú ræður hvernig síðan lítur út
Þú lagar síðuna að þínum þörfum. Stækkaðu eða minnkaðu myndir og texta með multi-level zoom til að sjá síðuna eins og þér hentar best í þínu námi.
Fleiri góðir kostir
- Þú getur prentað síður úr bókinni (innan þeirra marka sem útgefandinn setur)
- Möguleiki á tengingu við annað stafrænt og gagnvirkt efni, svo sem myndbönd eða spurningar úr efninu
- Auðvelt að afrita og líma efni/texta fyrir t.d. heimaverkefni eða ritgerðir
- Styður tækni sem hjálpar nemendum með sjón- eða heyrnarskerðingu
- Gerð : 208
- Höfundur : 6654
- Útgáfuár : 2014
- Leyfi : 380