Business Intelligence, Analytics, Data Science, and AI, Global Edition
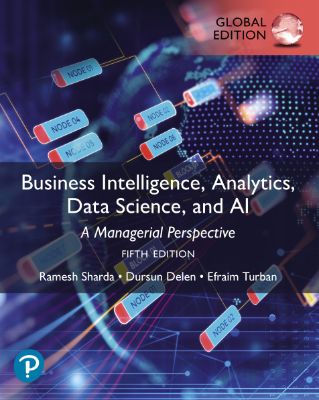
Námskeið
- T-707-VGBI Viðskiptagreind
-
V-785 – REK5
Lýsing:
Business Intelligence, Analytics, Data Science, and AI is your guide to the business-related impact of artificial intelligence, data science and analytics, designed to prepare you for a managerial role. The text's vignettes and cases feature modern companies and non-profit organizations and illustrate capabilities, costs and justifications of BI across various business units. With coverage of many data science/AI applications, you'll explore tools, then learn from various organizations' experiences employing such applications.
Ample hands-on practice is provided, can be completed with a range of software, and will help you use analytics as a future manager. The 5th Edition integrates the fully updated content of Analytics, Data Science, and Artificial Intelligence, 11/e and Business Intelligence, Analytics, and Data Science, 4/e into one textbook, strengthened by 4 new chapters that will equip you for today's analytics and AI tech, such as ChatGPT.
Annað
- Höfundar: Ramesh Sharda, Dursun Delen, Efraim Turban
- Útgáfa:5
- Útgáfudagur: 2024-08-23
- Hægt að prenta út 2 bls.
- Hægt að afrita 2 bls.
- Format:ePub
- ISBN 13: 9781292727530
- Print ISBN: 9781292459295
- ISBN 10: 1292727535
Efnisyfirlit
- Cover
- Cover
- Front Matter
- Title Page
- Copyright Page
- Preface
- Acknowledgments
- About the Authors
- About the Book
- Part I: Introduction
- Part I: Introduction
- Chapter 1: An Overview of Business Intelligence, Analytics, Data Science, and AI
- Introduction: An Overview of Business Intelligence, Analytics, Data Science, and AI
- 1.1: Opening Vignette: Sports Analytics—An Exciting Frontier for Learning and Understanding Applications of Analytics
- 1.2: Changing Business Environments and Evolving Needs for Decision Support and Analytics
- 1.3: Decision-Making Processes and Computerized Decision Support Framework
- 1.4: Evolution of Computerized Decision Support to Analytics/Data Science
- 1.5: A Framework for Business Intelligence
- 1.6: Analytics Overview
- 1.7: Analytics Examples in Selected Domains
- 1.8: Plan of the Book
- 1.9: Resources and Links
- Chapter Highlights and Key Terms
- Questions and Exercises
- References
- Chapter 2: Artificial Intelligence: Concepts, Drivers, Major Technologies, and Business Applications
- Introduction: Artificial Intelligence: Concepts, Drivers, Major Technologies, and Business Applications
- 2.1: Opening Vignette: Grant Thornton Employs Aisera Chatbot to Reduce IT Help Desk Burden
- 2.2: Introduction to Artificial Intelligence
- 2.3: Human and Computer Intelligence
- 2.4: Major AI Technologies and Some Derivatives
- 2.5: AI Support for Decision Making
- 2.6: AI Applications in Various Business Functions
- 2.7: Introduction to Robotics
- 2.8: Illustrative Applications of Robotics
- 2.9: Conversational AI—Chatbots
- 2.10: Enterprise Chatbots
- Chapter Highlights and Key Terms
- Questions and Exercises
- References
- Part II: Descriptive Analytics
- Part II: Descriptive Analytics
- Chapter 3: Descriptive Analytics I: Nature of Data, Big Data, and Statistical Modeling
- Introduction: Descriptive Analytics I: Nature of Data, Big Data, and Statistical Modeling
- 3.1: Opening Vignette: SiriusXM Attracts and Engages a New Generation of Radio Consumers with Data-Driven Marketing
- 3.2: The Nature of Data in Analytics
- 3.3: A Simple Taxonomy of Data
- 3.4: The Art and Science of Data Preprocessing
- 3.5: Definition of Big Data
- 3.6: Fundamentals of Big Data Analytics
- 3.7: Big Data Technologies
- 3.8: Big Data and Stream Analytics
- 3.9: Statistical Modeling for Business Analytics
- 3.10: Regression Modeling for Inferential Statistics
- Chapter Highlights and Key Terms
- Questions and Exercises
- References
- Chapter 4: Descriptive Analytics II: Business Intelligence Data Warehousing, and Visualization
- Introduction: Descriptive Analytics II: Business Intelligence Data Warehousing, and Visualization
- 4.1: Opening Vignette: Targeting Tax Fraud with Data Warehousing and Business Analytics
- 4.2: Business Intelligence and Data Warehousing
- 4.3: Data Warehousing Process
- 4.4: Data Warehousing Architectures
- 4.5: Data Management and Warehouse Development
- 4.6: Data Warehouse Administration, Security Issues, and Future Trends
- 4.7: Business Reporting
- 4.8: Data Visualization
- 4.9: Different Types of Charts and Graphs
- 4.10: The Emergence of Visual Analytics
- 4.11: Information Dashboards
- Chapter Highlights and Key Terms
- Questions and Exercises
- References
- Part III: Predictive Analytics
- Part III: Predictive Analytics
- Chapter 5: Predictive Analytics I: Data Mining Process, Methods, and Algorithms
- Introduction: Predictive Analytics I: Data Mining Process, Methods, and Algorithms
- 5.1: Opening Vignette: Police Departments Are Using Predictive Analytics to Foresee and Fight Crime
- 5.2: Data Mining Concepts and Applications
- 5.3: Data Mining Applications
- 5.4: Data Mining Process
- 5.5: Data Mining Methods
- 5.6: Data Mining Software Tools
- 5.7: Data Mining Privacy Issues, Myths, and Blunders
- Chapter Highlights and Key Terms
- Questions and Exercises
- References
- Chapter 6: Predictive Analytics II: Text, Web, and Social Media Analytics
- Introduction: Predictive Analytics II: Text, Web, and Social Media Analytics
- 6.1: Opening Vignette: Machine versus Human on Jeopardy!: The Story of Watson
- 6.2: Text Analytics and Text Mining Overview
- 6.3: Natural Language Processing (NLP)
- 6.4: Text Mining Applications
- 6.5: Text Mining Process
- 6.6: Sentiment Analysis and Topic Modeling
- 6.7: Web Mining Overview
- 6.8: Search Engines
- 6.9: Web Usage Mining (Web Analytics)
- 6.10: Social Analytics
- Chapter Highlights and Key Terms
- Questions and Exercises
- References
- Chapter 7: Deep Learning and Cognitive Computing
- Introduction: Deep Learning and Cognitive Computing
- 7.1: Opening Vignette: Fighting Fraud with Deep Learning and Artificial Intelligence
- 7.2: Introduction to Deep Learning
- 7.3: Basics of “Shallow” Neural Networks
- 7.4: Process of Developing Neural Network–Based Systems
- 7.5: Illuminating the Black Box of ANN
- 7.6: Deep Neural Networks
- 7.7: Convolutional Neural Networks
- 7.8: Recurrent Networks and Long Short-Term Memory Networks
- CHATGPT
- 7.9: Computer Frameworks for Implementation of Deep Learning
- 7.10: Cognitive Computing
- Chapter Highlights and Key Terms
- Questions and Exercises
- References
- Part IV: Prescriptive Analytics
- Part IV: Prescriptive Analytics
- Chapter 8: Prescriptive Analytics: Optimization and Simulation
- Introduction: Prescriptive Analytics: Optimization and Simulation
- 8.1: Opening Vignette: Balancing Delivery Routes, Production Schedules, and Inventory
- 8.2: Model-Based Decision-Making
- 8.3: Structure of Mathematical Models for Decision Support
- 8.4: Certainty, Uncertainty, and Risk
- 8.5: Decision Modeling with Spreadsheets
- 8.6: Mathematical Programming Optimization
- 8.7: Multiple Goals, Sensitivity Analysis, What-If Analysis, and Goal Seeking
- 8.8: Decision Analysis with Decision Tables and Decision Trees
- 8.9: Introduction to Simulation
- 8.10: Genetic Algorithms and Developing GA Applications
- Chapter Highlights and Key Terms
- Questions and Exercises
- References
- Part V: Software and Trends
- Part V: Software and Trends
- Chapter 9: Landscape of Business Analytics Tools
- Introduction: Landscape of Business Analytics Tools
- 9.1: Opening Vignette: How Seagate Is Using Knime to Tackle the Digital Transformation
- 9.2: Importance of Analytics Tools
- 9.3: Free and Open-Source Analytics’ Programming Languages
- 9.4: Free and Open-Source Analytics’ Visual Tools
- 9.5: Commercial Analytics Tools
- Chapter Highlights and Key Terms
- Questions and Exercises
- References
- Chapter 10: AI-Based Trends in Analytics and Data Science
- Introduction: AI-Based Trends in Analytics and Data Science
- 10.1: Application Vignette: Discover Foods Employs IoT and Machine Learning to Ensure Food Quality
- 10.2: Cloud-Based Analytics
- 10.3: Location-Based Analytics
- 10.4: Image Analytics/Alternative Data
- 10.5: IoT Essentials
- Major Benefits and Drivers of IoT
- 10.6: IoT Applications
- 10.7: 5G Technologies and Impact on AI
- 10.8: Other Emerging AI Topics: Robotic Process Automation (RPA)
- 10.9: Bioinformatics and Health Network Science
- Network Analytics
- 10.10: Other Recent Developments
- Chapter Highlights and Key Terms
- Questions and Exercises
- References
- Chapter 11: Ethical, Privacy, and Managerial Considerations in Analytics
- Introduction: Ethical, Privacy, and Managerial Considerations in Analytics
- 11.1: Opening Vignette: Lessons Learned from Analytics Journey in an Organization
- 11.2: Implementing Intelligent Systems: An Overview
- 11.3: Successful Deployment of Intelligent Systems
- 11.4: Implementing IoT and Managerial Considerations
- 11.5: Legal, Privacy, and Ethical Issues
- 11.6: Ethical/Responsible/Trustworthy AI
- 11.7: Impacts of Intelligent Systems on Organizations
- 11.8: Impacts on Jobs and Work
- 11.9: Potential Dangers of AI
- 11.10: Citizen Science and Citizen Data Scientists
- Chapter Highlights and Key Terms
- Questions and Exercises
- References
- Glossary
UM RAFBÆKUR Á HEIMKAUP.IS
Bókahillan þín er þitt svæði og þar eru bækurnar þínar geymdar. Þú kemst í bókahilluna þína hvar og hvenær sem er í tölvu eða snjalltæki. Einfalt og þægilegt!Rafbók til eignar
Rafbók til eignar þarf að hlaða niður á þau tæki sem þú vilt nota innan eins árs frá því bókin er keypt.
Þú kemst í bækurnar hvar sem er
Þú getur nálgast allar raf(skóla)bækurnar þínar á einu augabragði, hvar og hvenær sem er í bókahillunni þinni. Engin taska, enginn kyndill og ekkert vesen (hvað þá yfirvigt).
Auðvelt að fletta og leita
Þú getur flakkað milli síðna og kafla eins og þér hentar best og farið beint í ákveðna kafla úr efnisyfirlitinu. Í leitinni finnur þú orð, kafla eða síður í einum smelli.
Glósur og yfirstrikanir
Þú getur auðkennt textabrot með mismunandi litum og skrifað glósur að vild í rafbókina. Þú getur jafnvel séð glósur og yfirstrikanir hjá bekkjarsystkinum og kennara ef þeir leyfa það. Allt á einum stað.
Hvað viltu sjá? / Þú ræður hvernig síðan lítur út
Þú lagar síðuna að þínum þörfum. Stækkaðu eða minnkaðu myndir og texta með multi-level zoom til að sjá síðuna eins og þér hentar best í þínu námi.
Fleiri góðir kostir
- Þú getur prentað síður úr bókinni (innan þeirra marka sem útgefandinn setur)
- Möguleiki á tengingu við annað stafrænt og gagnvirkt efni, svo sem myndbönd eða spurningar úr efninu
- Auðvelt að afrita og líma efni/texta fyrir t.d. heimaverkefni eða ritgerðir
- Styður tækni sem hjálpar nemendum með sjón- eða heyrnarskerðingu
- Gerð : 208
- Höfundur : 8169
- Útgáfuár : 2024
- Leyfi : 380